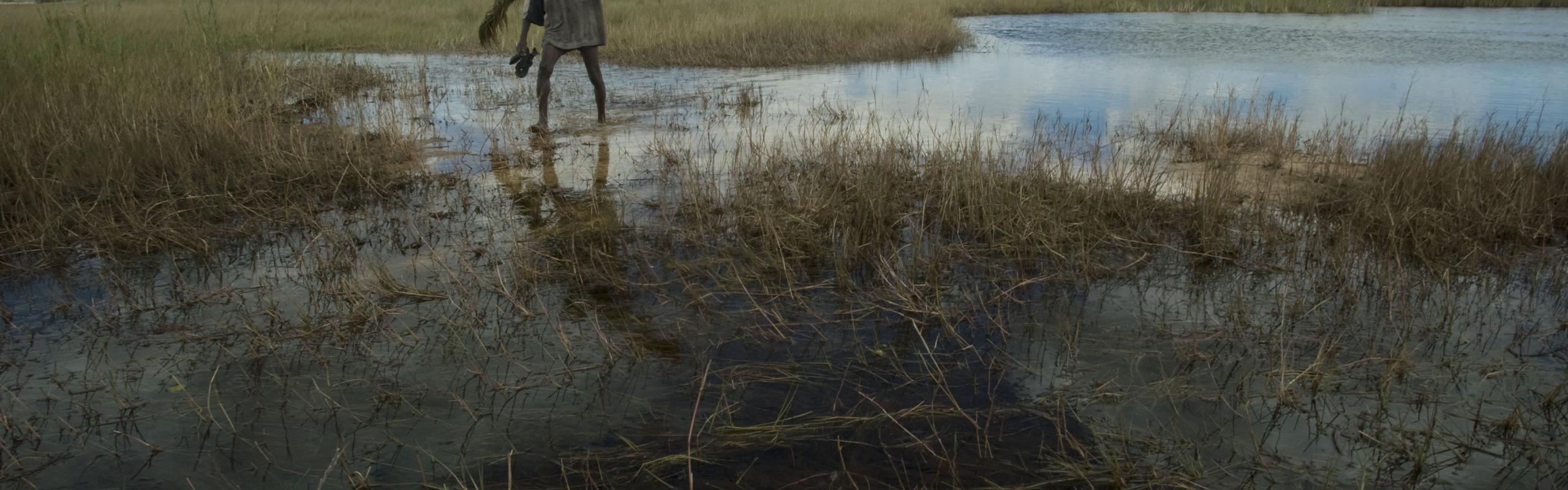
Climate change, disease outbreaks, price spikes, conflict - resilience to such shocks has become a widespread goal among development practitioners and policymakers, but what exactly is resilience? How can we define resilience and how can it be measured to ensure that programs and policies aimed at increasing poor populations’ resilience to shocks truly enhance food security and overall welfare?
To address these questions, the Food Security Information Network (FSIN) established a Resilience Measurement Technical Working Group (RM-TWG) to provide guidance on the establishment of sound resilience measurement practices. The latest publication in a series of technical papers from the Working Group looks at how to construct resilience variables and explore the relationships among those variables to conduct quantitative analysis for resilience measurement.
The RM-TWG has defined resilience as “the capacity that ensures stressors and shocks do not have long-lasting adverse development consequences.”[1] However, there also exist several other definitions of resilience in the broader literature that relate to the estimation frameworks discussed in the paper. The first involves the definition of resilience as a variable – the paper states that the capacity for resilience can be defined as a variable that is predicted, explained, or constructed by a selection of other variables. Second, resilience can be defined as the capacity that predicts individuals’, households,’ and communities’ well-being in the face of various shocks. Third, resilience can be defined as the property of a well-being outcome.
After discussing the definition of resilience as a concept, the authors move to discussing which quantitative methods can be helpful in defining a set of indicators to measure resilience. The authors argue that resilience is multidimensional, being composed of various context- and time-specific dimensions. However, resilience can be represented as clusters of indicators, each of which can then be measured separately. The selection of which dimensions should be used to estimate resilience must be based on both empirical evidence and specific contexts.
They also mentioned that resilience is a latent variable or sometimes, a unobserved variable (the characteristics and factors that make up resilience may not be observable).
Efforts to aggregate resilience’s various dimensions into one single measure demands the use of an index that allows for more concise descriptions of the concept and can help improve comparability, ranking, targeting, and aggregation of resilience measurements across settings. However, as with any index, aggregation is needed and this can be done with a variety of assumptions, some try to define the role of each variable in the index, while others will not look at each individual variable. The paper goes on to highlight several examples of analytical methodologies that support each of these principles. These include factor analysis, principle component analysis (PCA), Structural Equation Models (SEMs), and Multiple Indicator Multiple Causes (MIMIC) models.
In terms of estimation methodologies to explore resilience relationships, the paper discusses the need to formalize these relationships. That begins with estimation of the resilience variable or index as part of multivariate model, taking into consideration possible sources of bias. Estimating this relationship will also need to consider non-linear effects or clustering and will require the data to determine the shape of these effects and the possible need for a multi-level model.
Regarding data requirements, the paper suggests that household surveys, particularly nationally representative datasets, can be used to estimate household resilience. Households’ resilience can be estimated using either a static or a dynamic framework. In a static framework, cross-sectional data can be used such as Living Standards Measurement Surveys that include questions on income, income-generating activities, access to basic health and education services, social networks, safety nets, productive and non-productive assets, and living conditions. On the other hand, dynamic frameworks require longitudinal, panel, or pseudo-panel data. Regardless of the type of framework, household datasets should be complemented with community-level or ecosystem data.
While the authors do not discuss the specifics of the studies, they do also present several examples of recent studies that have been conducted using the quantitative frameworks laid out in the paper. These include FAO’s work in Somalia and USAID work in Ethiopia .
[1] Constas, M., Frankenberger, T. & Hoddinott, J. 2014. Resilience Measurement Principles: Toward an agenda for measurement design. Rome: FSIN (FAO/WFP).
By: Sara Gustafson, IFPRI