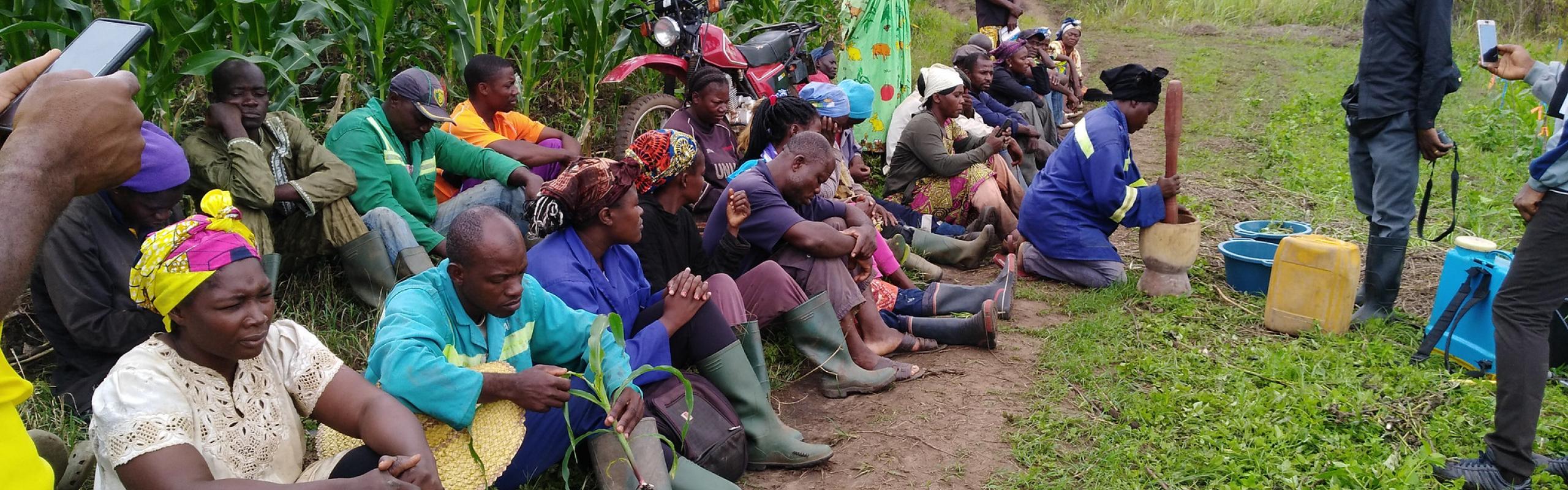
Written by Joe Glauber, Senior Research Fellow in IFPRI's Markets, Trade and Institutions Division, and Seth Meyer, Professor at the Department of Agricultural Economics from the University of Missouri.
Crop production statistics are an important asset for the commodity markets around the world. Important decisions based upon crop production estimations are made around the clock by different actors in the commodity markets, such as production, trade, storage, and food aid, among others. More accurate and timely information on crop production improves market functioning and enhances market transparency. Transparency in turns benefits producers and consumers.
As an example, let’s consider how information regarding crop size helps resolve uncertainty in the US soybean market. Ending stocks are a much-watched indicator of available supplies in the market. Stocks and price work in opposition to ration use and ensure supplies.
In late September of each year, the US Department of Agriculture (USDA) releases its estimates of the quantity of old crop soybeans held by farmers, processors and commercial storage operators as of September 1. These stocks reflect an estimate of the amount of supplies carried over into the next marketing year. Ending stock can be expressed as an accounting identity that is equal to supplies available (ending stocks from the previous year plus production plus imports) minus whatever is consumed or exported that year:
Ending Stocks (year t) = Ending stocks (year t-1) + Production (year t) + Imports (year t) – Domestic use (year t) – Exports (year t).
USDA produces its first forecast of US (and global) soybean ending stocks in the May World Agricultural Supply and Demand Estimates (WASDE) report, roughly 17 months before those estimates will become finalized. Over that period, information is gained regarding the size of current year carryout, the size of the US crop, and all the major sources of demand such as size of domestic crushing, seed use, and exports.
Figure 1 shows the forecast accuracy of the USDA estimate of US soybean ending stocks based on the root mean squared error (in metric tons) taken from the previous 20 years of WASDE estimates. (The root mean squared is a statistical measure that measures the accuracy of the forecast relative to the actual value, in this case the “final” forecast estimate.)
Not surprisingly, the forecast errors for ending stocks (but in fact all supply and demand variables) are highest in the early months of the forecast period, when little is known about supply and demand factors as the US crop is just going into the ground. As the forecast month gets closer to October of the following year (that is, the first month after release of the September 1 ending stock estimate), the forecast error for US soybean stocks falls to essentially zero. Note that the forecast errors do not fall in equal increments across time but fall when some uncertainty about the market is resolved. For example, figure 2 shows that forecast uncertainty concerning the size of the US soybean crop is largely resolved over the period August-October. More certainty regarding the size of the US crop helps contribute to more certainty regarding the size of ending stocks (as evidenced by the smaller forecast errors)
Likewise, forecast uncertainty for US ending stocks is tied to demand factors such as exports. Brazil is a major competitor of the US in global export markets so the size of Brazil production can have an effect on projected US exports and ending stocks. Forecast uncertainty for Brazil soybean production is typically resolved over the months of December to April. As the marketing year progresses, more and more is known about the supply and disposition of the crop and forecast errors for ending stocks decline rapidly over April to September.
What is the value of a more accurate forecast? With an inaccurate forecast, quantities are misallocated over time and space due to inefficient price signals, resulting in social welfare losses. For example, poor information on crop production in major production regions can result in a sudden, unexpected need for imports in the event of a drought, or a surge of exports in event of a bumper crop. Improved information on production can be critical in food insecure areas prone to drought like the horn of Africa.
While countries like the United States have long benefited from extensive survey-based data collection, information regarding production data in many developing countries has been subject to considerably more uncertainty. In recent years, with the spread of open data, new satellites and sensors, and open access to various satellite imagery and products, Earth Observation (EO) data is taking a bigger role helping to improve crop production estimations. Timely and publicly accessible information helps to understand the situation for different crops globally, improving also crop estimations accuracy and timeliness. Variables such as evaporative stress index, soil moisture, temperature, precipitation, normalized difference vegetation index (NDVI) among many others, are strong indicators that help analysts around the world to have better estimations of crop production. When combined with other data, including traditional survey data, meteorological data and expert input, these earth observation data help produce better estimates more quickly, and those EO data are only going to get better over time.